Background
At Pontem Health we are always looking for ways to process data in ways that allow individuals and health care professionals to reach clear conclusions, as well as predict future outcomes based on predictive models. This includes daily living, as well as recovery from surgeries, and optimizing fitness routines. We decided a fun way to highlight the usefulness of analytics was to try and predict my first marathon finish time based on data generated with my smart watch during my training. Additionally, it seems it is the fall marathon season and my friend and colleague Asher Mortimer is running a marathon in October, and has been collecting his training data to share. So, look for more posts in this series on health analytics.
I recently ran in the Equinox Marathon in Northern Colorado, which I was reluctant and nervous to do given I hadn’t run one before. I decided the only way to do this properly was to go full-tech mode and use my smart watch data to drive my training. It seems everyone has a fancy watch that puts out unbelievable data, yet I had a hard time finding people who did too much with the data. What a perfect opportunity to discuss what I did and figure out what data tends to predict the closest outcome compared to the final result.
The data collected is nothing short of amazing. Not only will it track your heart rate and how far you run, but it will provide elevation changes, power, stamina estimates, training effects, and a ton of running dynamics data, including: run cadence (steps/min), stride length, vertical ratio, vertical oscillation, average ground contact time. It really is enough to make one’s head spin. My hope is to make some sense of all the data and show what worked best for me while training.
After signing up and getting over the “I just want to finish” attitude, I set a goal to finish in sub-4 hours. Given my age (50), yet fairly active lifestyle and I have run a half marathon in less than 2 hours, I figured that was a good goal. I found processing the data generated from all my training was more complicated than anticipated, and smart watches in general can be…optimistic about predicting results. Check out the table below comparing what my watch predicts for several races. Presumably, the times generated are based on some wholistic view of my training, however I could not figure how it reached the conclusions it did. For comparison, I’ve listed my previous personal bests in the three races I have run. Granted, I never trained as intensely as I did for this marathon, at the same time many of my PR’s were set by a younger version of me (3-5 years ago). Five years is the better part of a career for several professional sports!
As I did my stretches at the start line, I had visions of absolutely crushing my 4-hour goal based on what my watch was telling me I could do. Disclaimer: I did get an email from my smart watch claiming the next software update in September would feature improved race prediction algorithms. I did accept the push prior to my race and assume these numbers are based on the improved predictor.
Look at Mikey, I think he likey, we are sinister sons
It shouldn’t surprise people that I chose a race with several challenges. The Fall Equinox Marathon starts at 6,339 ft elevation and has about 1,000 ft gain over the first 5 miles. Not only that, there are some severe downhill sections where the grade reaches -13.7%! All in, runners climb almost 1,500 ft and descent 2,600 ft by the time they reach the finish line. And just when one is gassed, the last 1.2 miles has some slight uphill gradients and the overall downhill slope is much less than the previous 10 miles. This feels like pulling a plow!
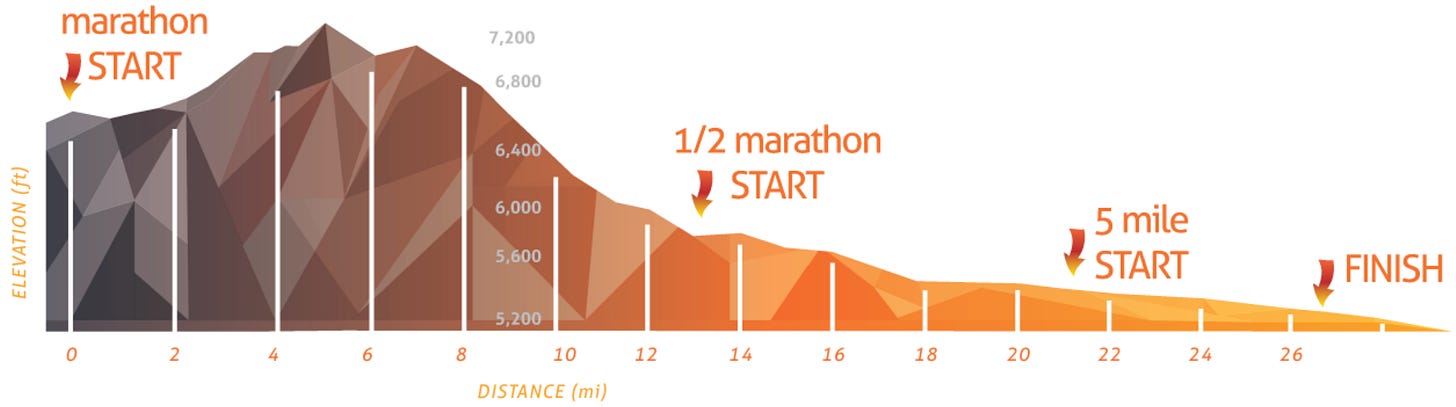
How you like my stylin’, bruh? Ain’t nobody stylin’ bruh
Let’s get into the data! Training for this race, I ran 3 or 4 days per week in several formats: sprints, trail runs, tempo runs, and long runs. From June 1 thru September 17, I ran just over 460 total miles. The younger me would have just put in the time. But I’ve learned how you run matters and the training program matters, too. We’ll make a couple of comparisons from some of my runs to highlight some of the analytics used during the training, and then we’ll take a look at my fitness progression to see if we can make some predictions on how my finish time will look. Of course, if you just want to see how fast I ran, scroll to the bottom and see if I met my expectations.
Sprints: Checking for Vertical Oscillation
My weekly workout program included one anaerobic training session: sprints. While people think about endurance when they think about marathons, it is also important to continue to develop muscle strength while training. Additionally, I knew I’d be challenged with hills early in the race and would need the anaerobic fitness to manage that phase of the race. What exactly did I look at when I ran these sessions? One example is highlighted in the following routine: I warmed-up with a 10-minute jog and then I performed 10 x 400 m sprints with a 1:30 recovery jog between each set, followed by a 5-minute cooldown. In the chart below, the dark blue area is my pace (mm:ss, left axis) and the dots represent my vertical oscillation (cm, right axis). Vertical oscillation describes how much one is bouncing up and down during their stride. The theory is effort should be made in moving forward; too much vertical movement indicates the energy isn’t being used to propel the runner forward. In this workout, I maintain a pretty consistent oscillation, but I do start showing signs of fatigue after round 7. My pace starts to dip and the oscillation is more erratic.
Later that week, I ran an 8-mile tempo run and a 20-mile long run. I came back to sprints the following week, and ran 8 x 800 m sprints with the same 1:30 recovery lap. During the sprints, my oscillation wasn’t too different from the previous week. However, I was able to maintain good form throughout the routine. Even during the last spring, I was able to push faster for a good finish, and ran smoother with less bounce.
This analysis helped me understand my anaerobic fitness was improving and led me to try and increase my pace during long runs.
Tempo Runs: Power As A Metric For Efficiency
Smart watches offer insights on your total effort through power (Watts) calculations. Previously, it often required a secondary device in your shoe but now it does it all singularly from your wrist. Power data can provide more immediate feedback allowing athletes to train more consistently. Pace and heart rate can have a delayed response, whereas power data will account for vertical, lateral, and horizontal acceleration of your foot while running; factor in wind, terrain, elevation changes, and altitude. The overall benefit is it can help regulate ones energy during runs. The software calculates power in real time and tells the user how hard they’re working regardless of the terrain.
One routine I ran 7 weeks apart, involved a 2-mile warm-up followed by 3 miles run at short tempo pace [~7:40 min/mile (4:46 min/km) pace for me] followed by a 1-mile easy pace. In the first run (top chart), I wasn’t hitting my desired pace (beginning around 18 minutes) and I kept notching up the pace until I finally hit it during the final mile. The interesting thing you’ll notice is my pace was increasing while my power was staying relatively constant. While the chart doesn’t show elevation, there is a gradual decrease in elevation beginning just before the 30 minute mark. By holding the same effort, a faster pace resulted from the decline in elevation. My average power was 376 W with a max of 508 W (t = ~14 minutes) to get up a steep hill. In comparing the same run on the same course, the results show an improved strength and endurance. While I had to navigate some traffic, I was better able to reach and maintain my pace, even exceeding it during the last mile. The faster pace was achieved with lower power: average power was 368 W with a max of 466 W (the same hill was climbed more easily). Given my race was a week and a half away from the second run, I felt my fitness level was peaking and I was understanding how much effort I needed to put into my desired pace.
Predicting My Finish Time
What’s More Effective: Take Bigger Steps Or Step Faster?
To get from start to finish faster, one can take fewer steps (bigger strides) or take steps faster. When running a race, does it really matter how long your stride is? Maybe. If your stride length is 1 meter, you will take 42,195 steps in a marathon. However, if your stride is increased to 1.1 meters (less than 4 inches!) you will take 3,836 fewer steps. My normal walking gait takes about 2,300 steps to reach a mile. A big stepper will effectively shorten the race! On a macro level, that alone will decrease your finish time!
I tried putting that to practice. Below, are my monthly run totals along with average stride length data and run cadence averages (steps per minute). While I started training in June, I added April and May when I wasn’t training for comparison. During the summer, I had steady increases in stride length (teal line), however as I moved into peak and then taper training modes in September, my stride length dipped. My cadence (steps per minute) also increased. Will either of these accurately predict my race time?
Well, if you look at the matrix from my training and I keep my stride and cadence in line with my training runs, I should be able to beat my goal! In reality, the difference between June and August stride lengths was only 3.5 inches. That seems too fine to focus on when running 26.2 miles. This matrix shows roughly a 40 minute performance window with an average time of 4:06:37. If I claim I’ll hold the gains, the average time for Aug/Sept data improves to 4:00:11. It’s important to note here that none of the times calculated here come anywhere near what the smartwatch was predicting (3:30:44).
I can’t even relax without sirens off in the distances…
It feels like a more impactful way to cut race times down would be to focus on how quickly you’re picking ‘em up and putting ‘em down. Experts will say a cadence of 180 spm is an optimal rate for running efficiency. Obviously, I never got there, and if I ever do this again (there may be some Pontem competition brewing…) I will probably focus a little more on trying to get to that optimal cadence. So, looking at several cadence/stride combinations to achieve a 4 hour race time shows within half a footstep the cadence can fluctuate 23 spm.
Does anyone want to put money on the 4 hour over/under line?! Is there more to it than how far you step and how quick you make those steps?
Can I Keep A Fast Enough Pace Without Exhausting Myself?
One of the most common metrics when running long distance races is pace, or minutes per mile. Not only does running mechanics influence running pace, but overall fitness plays a large role, too. There are several fitness metrics to rely on, such as heart rate or VO2 max. Let’s look at heart rate. Two very distinct trends show up when looking at my marathon training: I was able to improve my pace (shorter time per minute) as I moved through my training, and my average heart rate dropped over my training cycle. In May, my average pace was 9:51 min/mile (6:07 min/km) compared to a much faster pace of 8:43 min/mile (5:25 min/km) in September. I am oversimplifying the analysis slightly, because my pace was intentional during my long runs. I purposely ran at pace slower than my desired marathon pace in a progressive fashion: setting a faster pace within each month.
I shaved almost 50 seconds per mile off my time, which was by design in my long runs and one may cynically say I was just running faster/harder. But my average heart rate dropped from 147 bpm to 139 bpm, meaning I was getting in better shape. My August heart rate average jumped up almost 3 bpm because my long distance runs increased dramatically: I ran 3 20-mile distances, and I was able to continue to improve my pace despite the taxing distances. The biggest gains in pace were made in August, when my average pace dropped below a 4-hour race time. My average pace in September puts me on target to finish close to 3:48:00, which is still almost 18 minutes slower than what my watch was predicting! However, this is in line with the fastest pace predicted using cadence and stride length.
When talking about heart rate data, one is really talking about endurance. The key to the training is to be able to do two things: maintain an elevated heart rate for a long time AND to be able to run without having your heart rate get too high to the point of exhaustion. The chart below compares distances run and average/max heart rates. As one would expect, as distances increased, my average heart rate increased. The distances between 13 - 18 miles also resulted in the highest max heart rate. Something different happened in my 20-mile runs: my average and max heart rate decreased. I purposefully paced myself to finish these longer runs.
My average pace for the 20-mile runs turns out to be 9:08 min/mile (5:40 min/km). Maintaining that pace will put me across the finish line approximately at 3:59.
There’s another aspect to fitness, which is VO2 Max levels. Stay tuned in for a deeper dive into that and other running data analyses when we catch up to Asher Mortimer next month after he runs his marathon! By the way, I would not bet against him beating my times!!
So, what do you think is the biggest driver to a marathon finish time? What would you pay most attention to if you had to guess when I’ll finish?
Of course, you can always guess the course will eat my lunch and I’ll never get anywhere close to these times!!
The vision becomes a vow to seek vengeance on all the vicious
Post-Race Reactions
So, how’d it turn out? I was happy to have made it to the finish line, and even happier to have finished right at my goal: 4:00! It seems Endurance was the winning category to guess my race time. My time didn’t come without some highs and lows. Let’s review what happened.
As my kids say, “You were cooking.” Until I wasn’t. The chart below shows the course elevation and my average pace for each mile. In the 19th mile, I experienced my first cramp. If I had been able to maintain the 8:45 min/mile (5:26 min/km) pace for the last 8.2 miles, I would have finished with a time of 3:48, matching the Pace metric.
Things got progressively worse after my first leg cramps, all the while I was slugging water, electrolytes, and carbs to recover. I restarted my running mix with Kickstart My Heart and a steady dose of Lil Wayne, AC/DC, Kid Rock,Volbeat, AfroJack, and Run Them Jewels Fast kept me moving forward, but not very well at all!
At mile 24, the 4:00 pacer caught up to me, and that fired me up enough to embrace the suck and get over that finish line (in truth, Strava clocked me at 4:00:06, but close enough)! Out of 146 marathon runners, I finished 46th and placed 4th in my age bracket.
With all this new technology out there, the data can become fairly complicated and it isn’t intuitive to try and use it to reach peak performances, let alone hold gains, or achieve goals. Hopefully, my training journey gave you some good ideas, and if you’ve got some health related data science, be sure to reach out to us! And be sure to be on the lookout for Asher’s post on his marathon experience, where he will look at other aspects of the complicated world of running analytics!