Case Study: Optimizing Chemical Delivery
Logistics Optimization Through Adaptive Multi-Agent Spacing
Our latest case study at Pontem Analytics is another example of our vision of bridging the gap between domain knowledge and data analytics. We are excited to share these examples as we think it will resonate with any company involved with logistics of product distribution. In this example, we have successfully implemented a data-driven, AI-powered logistics optimization model to enhance chemical delivery efficiency across vast oilfield networks. This is a complex problem because there are so many variables that can appear seemingly independent, yet at-times counteracting. It is not solving a simple maze to create the shortest roundtrip route while hitting all locations. Each location might will have different chemicals to deliver and each tank will be a different size. The chemicals will be consumed at a different rate. If a delivery truck arrives on a remote site and one tank is 70% full, it seems making the long trip is a waste to only “top off” the tank. But, perhaps if it isn’t filled then the tank may run empty before the next scheduled delivery. It seems optimizing the timing to allow the tank to drain nearly empty is easy. But multiply that by 100 locations and factor in the cumulative distance between delivery locations, and the problem becomes quite complex. Coming to an optimized solution without advanced analytics or AI tools is almost impossible.
In our chemical distribution example, we’ll share howe we integrated advanced forecasting, adaptive scheduling, and dynamic routing to reduce costs, lower emissions, and improve operational safety.
Challenge: Managing a Vast, Remote Chemical Distribution Network
One of the biggest challenges in onshore oilfield operations is managing a network of hundreds to thousands of production wells, each requiring precise chemical injection. Traditional delivery scheduling often results in inefficiencies due to:
Scattered well pad locations: Spanning hundreds of miles to locations that are often remote creating isolated and inefficient route options.
Variable consumption rates: Different tanks require refills at unpredictable times.
Tank size variability: Each site has unique storage capacity constraints.
Delivery scheduling inefficiencies: Static routing models lead to unnecessary travel, increasing costs and emissions, all which make for an unattractive HSE profile.
Remote tank telemetry has improved monitoring with real-time tank level alarms, but the logistics of chemical delivery remain an operational bottleneck. Tank telemetry technology was a game changer because it reduced massive demands on manpower for remote, unmanned well pads. Previously, an operator needed to send someone out to check on all tank levels to ensure pumps were working, tanks were filled with chemical, etc. If someone only visited a site once every two weeks and noticed a tank was close to half empty, an order would be placed just to avoid going empty. Tank monitoring systems introduced some safeguards to avoid this problem. Programmable notifications can be set such that once a specific volume is reached, an alert is automatically sent to appropriate personnel. This does solve many problems, however problems can still arise when forecasting out one to two weeks out delivery routes, volumes, etc. If a delivery notification is received after schedules are made, then emergency runs need to be made, which creates inefficiencies. There are, of course, work-arounds to ensure a larger safety margin is in place to avoid emergency runs. However, when servicing vast networks the safe guards can be inefficient. Not only that, it is often the case that some tanks will have monitoring tools installed and some will not. This creates complications and risks becoming too dependent on one notification to drive the needs of multiple unknown locations.
Our Approach: AI-Driven Adaptive Logistics Optimization
In order to be robust and agnostic to technologies in place, our process needed to be flexible enough to include features important to clients, and also adaptable to multiple platforms / industries. We started from a simplistic forecasting model to create a baseline scenario. We fixed the system on a recurrent neural network (RNN), which allows the forecasting models to process sequential data. We chose this route because these systems are good at capturing long-term dependencies in data. In other words, factoring in all the various chemical tank order frequencies.
Our next step was to allow these networks to talk to one another, as we understood each well pad was its own ecosystem, so to speak, yet for delivery purposes we needed to connect it to the overall delivery network.
With a rough cut-out in place, we relied on reinforcement learning to optimize the process, and brought in standard logistics assistant to help automate the process. Lastly, many of the delivery sites, could be served by multiple warehouses or delivery routes. The integration process was instrumental in creating a seamless system that allowed platforms to communicate properly. The schematic below highlights the development process just described.
Key Implementation Details & Results
To validate our methodology, we employed three major operating regions, analyzing over 1,000 tanks with varying consumption patterns and constraints. Our AI-driven model continuously learned and adapted to these variables in real-time, leading to measurable improvements in logistics efficiency. The results were groundbreaking:
20% reduction in delivery costs due to optimized routing and reduced fuel consumption.
15% improvement in fill efficiency, minimizing wasted trips and maximizing chemical utilization.
By eliminating inefficient detours and emergency deliveries, we reduced overall miles driven and unnecessary idling, resulting in a 30% cut in CO₂ emissions—a major win for both making roads safer and environmental impact.
Our system continuously adapts to operational changes in real time—whether it’s a sudden shift in demand, a road closure, or an equipment failure. By proactively adjusting schedules and routing, we’ve enhanced supply chain reliability and responsiveness, reducing the need for costly last-minute adjustments.
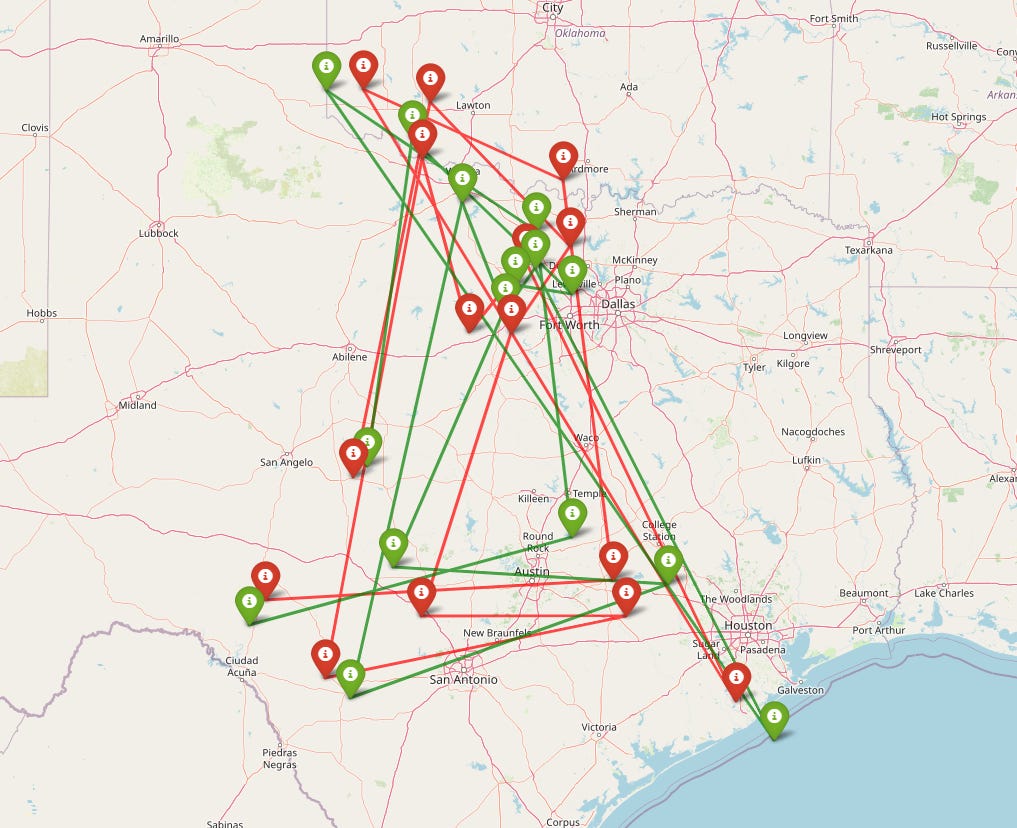
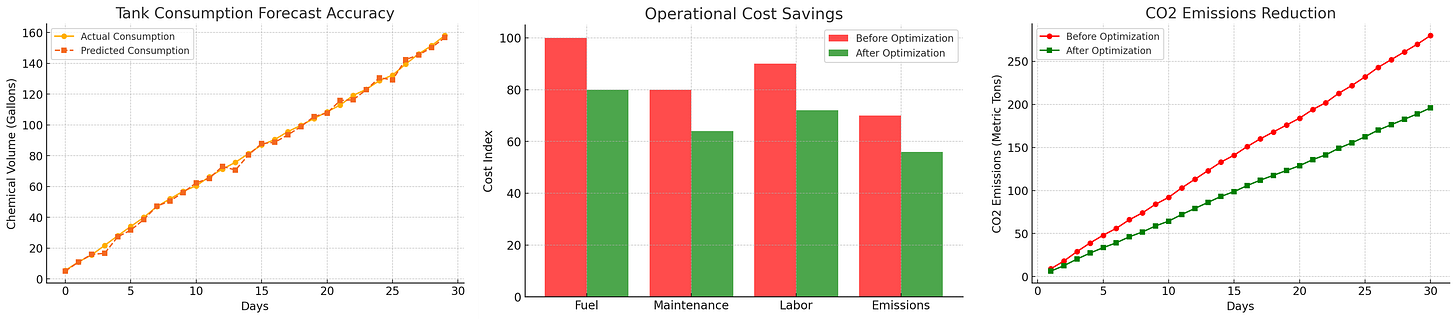
The Future of Logistics Optimization at Pontem
Our work has shown that AI-powered, data-driven logistics solutions are not just theoretical but tangible, scalable, and operationally viable. As we continue refining our models, we are actively expanding into:
Automated dynamic scheduling to further enhance real-time decision-making. These features are especially enticing considering operations impacted by emergency orders, rush scheduling, and/or fleet maintenance challenges. Having a real solution to overcome these unexpected, yet routine, inefficiencies is a massive win.
Self-adjusting tank thresholds to dynamically balance cost and efficiency. For example, if a delivery location begins to record improved delivery response time, then adjustments can be made to allow on-site supplies to be lowered before an order is made. The lower delivery stops on a monthly or annual accrual will result in significant savings.
Broader applications in supply chain logistics beyond oilfield chemicals.
Cross-Industry Applications
The same AI-powered logistics optimization methodology can be applied to various industries where logistics, delivery, and supply-demand balancing are critical. This includes crude logistics, where AI improves transportation efficiency from extraction sites to refineries; LNG offloading, where optimized scheduling minimizes delays at ports and storage facilities; industrial gas distribution, ensuring timely medical and manufacturing gas supply; agricultural chemical supply, where streamlined fertilizer and pesticide distribution reduces waste and enhances supply chain efficiency and many more.
By leveraging this approach, companies across the energy, industrial, and agricultural sectors can significantly enhance operational efficiency, reduce costs, and create more sustainable supply chains. If you’re interested in leveraging this technology in your operations, let’s talk.